Messy data? Here’s how you can still be data driven.
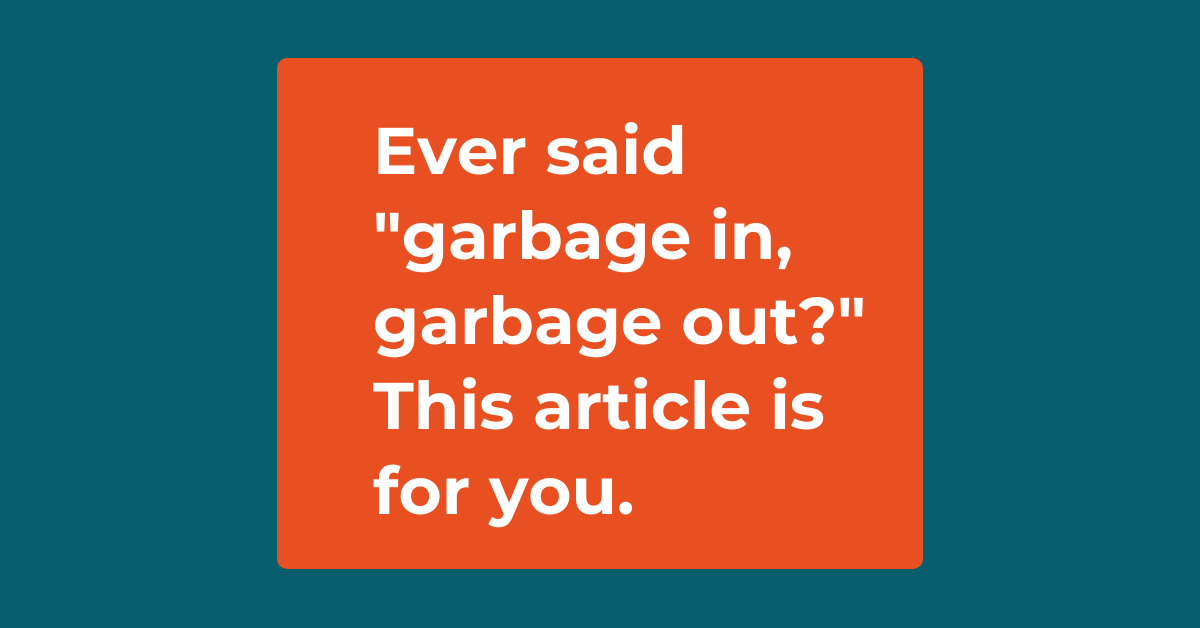
Having worked with customers ranging from hyper-growth startups to Fortune 500 companies, I can tell you every company says their CRM and marketing data is a complete mess.
Falkon is a revenue analytics platform that reasons over this data, so hygiene comes up a lot. We go beyond the top layer of data to find insights in the iceberg. When we start talking about that, ops and analytics teams consistently tell us they have a garbage in, garbage out problem.
Here’s what I don’t understand about that statement: If the data is so messy that it’s useless, why are you using it to run a company?
Clearly, the data must be worth something. The mindset that it’s not prevents companies from being truly data driven. It leads to a cycle like the following.
- Let’s do a big data cleaning project.
- In 3 months we’ll have good data.
- Then we can start using it.
- Until then, use the handful of dashboards that analyze less than 1% of data we collect.
Here’s how the project actually pans out:
- No one can agree on the right fields and structure.
- Data cleaning project takes 6 months.
- Data is still not clean.
- AEs and marketers just want to get their jobs done, so they’re not invested in the data cleaning project.
- Data gets messier.
Rinse and repeat. I don’t think such projects come from a bad place. If anything they come from people’s deepest wish that their data be pristine and clean, and I can certainly understand that wish. My own wish is that we start approaching messy data differently.
A better approach to messy data
A better approach starts with a radically different mindset. Here’s something from Ray Dalio on that.
Accept the following reality: Your data will never be “clean.”
Embrace the reality that data will always be messy and cleanliness is not a destination or a one-time project. Just like meditation or a healthy lifestyle, data hygiene is a lifelong practice.
Once this frame is in place, meaningful progress towards being data driven can happen. And believe it or not, you can get there through three straightforward techniques.
Three steps to become data driven
#1 Purpose-driven, three-day “cleaning” iterations
Pick one scoped and specific business problem you’d like to solve. For instance, let’s say your organization really wants to tackle the problem of having too many past-due renewal accounts. Do a three-day sprint (and no sprint should ever exceed five business days) to clean the one to three fields that will provide 90% accurate insight into accounts that have upcoming contract expiration dates. Day one is about identifying the requisite fields and how they need to be filled. Days two and three are about historical cleanup for existing accounts. Federate this to account owners and provide an incentive—an Amazon gift card or public acknowledgement goes a long way.
#2 Daily rituals & reinforcement
Once the sprint is over, you’re in good enough shape for the few fields that solve the specific business problem you were going for. Set up an automated feedback loop for owners of these fields to keep them up to date. This loop must be automated because no one person can nag consistently. In the example above, you could set up a weekly alert that goes to every account manager and their manager. It can also feed a leader scoreboard of team members who are in great shape, team members who are most improved, and ones who are lagging behind. This kind of transparency drives accountability. Social proof and oversight are incredible emotional reinforcement. And remember to celebrate the wins. Positive reinforcement tends to be more durable in the long term.
#3 Focus on what’s important
Not all fields are born equal. Data ultimately exists to serve business decisions. It has no innate value. It’s essential that “cleaning” sprints and daily rituals only be applied to data that actually give business leaders the visibility they need to make better decisions.
So what’s important? The answer will be different depending on your business and goals. Here’s an example, eight common sales fields we recommend for high cleanliness to our hyper-growth SaaS customers.
8 data fields to clean for pipeline visibility
- Segment: Many businesses are going up market. Limited visibility into segments dramatically reduces your ability to evaluate and forecast the pipeline mix.
- Industry or persona: Understanding conversion rate by industry or persona helps you understand your most promising segments and build a pipeline that is likely to have higher conversion rates.
- Opportunity stage: So many opportunities are stuck being counted in the wrong stage. This leads to an inflated sense of pipeline.
- Expected value: Again, it’s easy to think you have a more robust pipeline than you actually do by inflating expected deal size.
- Expected close date: Yet another way to accidentally create an optimistic pipeline view.
- Contract end date: Critical for rigor around reaching out to customers about renewals in advance and getting everything sorted out BEFORE the renewal date.
- Source: Where is pipeline coming from? Accurate source data helps you identify and bet on ones with high conversion rates and faster deal velocity.
- Activity: It used to be difficult for reps to log all activity manually. However, now with a lot of sales workflows being augmented with technology, activity via email / phone / meeting can all be automatically logged to help create a view of the customer journey.
What will you wash?
As you consider your own data reality, remember that a good data hygiene practice serves your business. Small efforts add up, so even if you focus on one data set, you can enable better, faster business decisions if you recognize your data will never be totally clean, and then wash the bits that matter most.
Want more articles like this? Follow Falkon on LinkedIn >